This is why the AI skill gap is not just a question of tech capability
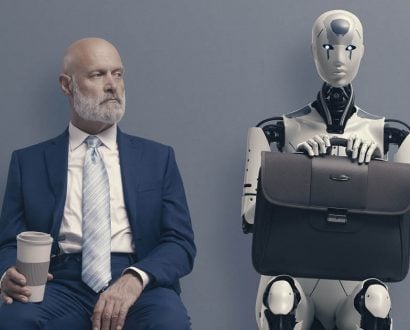
Australian businesses, like those around the world, are struggling to find good tech talent. Homegrown skills are hard to find and it’s a tough time for bringing in expertise from overseas. This issue is broadly true in the sector, but even more so in AI.
And with AI driving a major new wave of transformation initiatives, it’s a problem that can undermine efforts at a critical phase of adoption and integration.
But before we spend too many resources hunting for AI coders and data scientists, there’s another skill gap we need to solve in the executive team.
Aligning with objectives
No serious tech initiative succeeds without a plan. To know why we should adopt AI and what exactly it can do for us, we need to know how it will align with our business objectives and help us to improve our ability to deliver on our goals.
If business leaders are struggling to understand what is truly possible using AI tools and platforms, and the risks that must be managed to achieve successful integrations, all the tech talent in the world won’t be enough to build a solution that truly works for our needs.
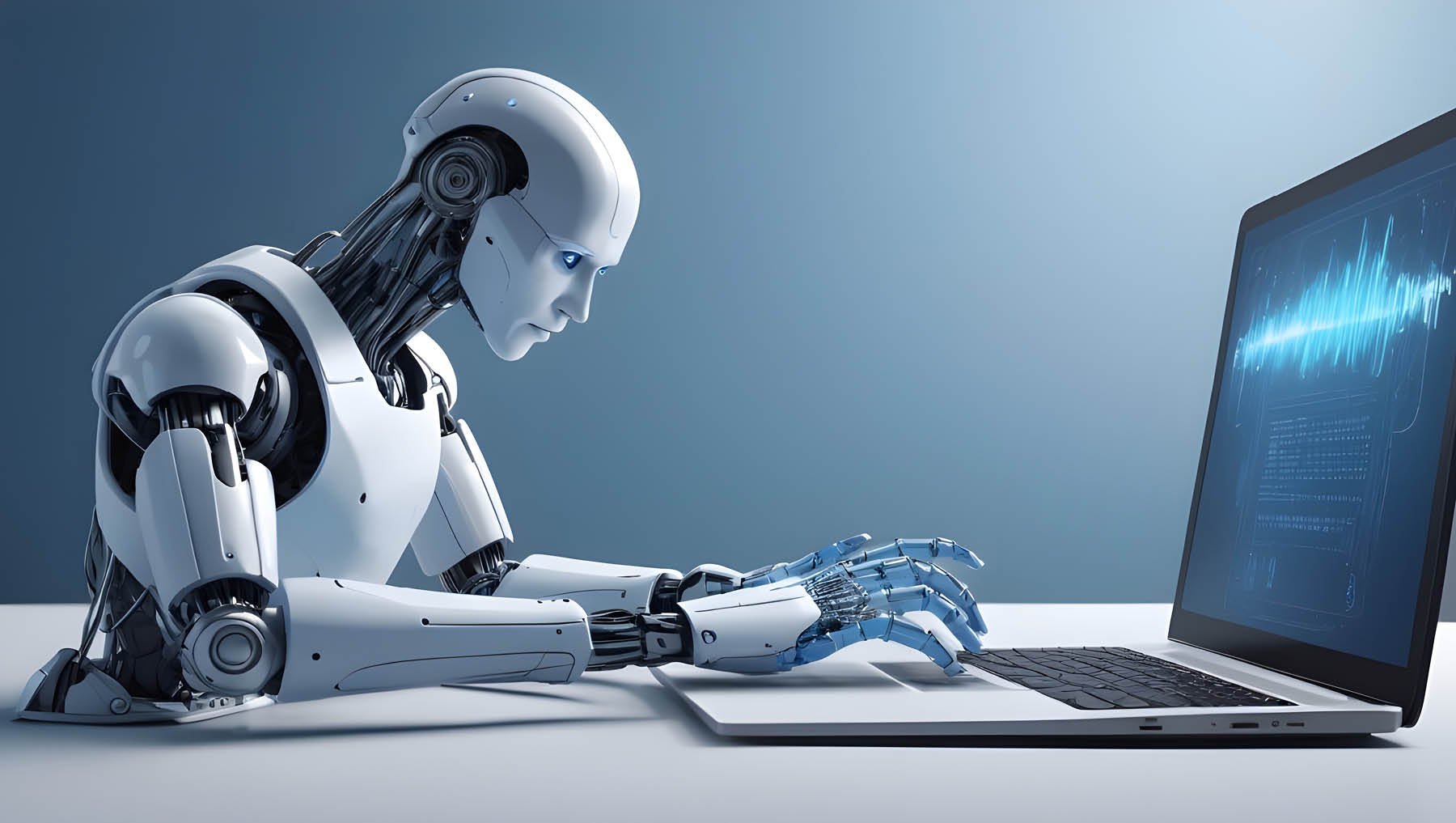
False confidence and a fear of missing out can be a dangerous cocktail.
The new IDC ‘Data and AI Pulse: Asia Pacific, 2024’ study, commissioned by SAS, shows AI spending is set to rise rapidly from US$45 billion in 2024 to US$110 billion by 2028. While this indicates a strong belief in the potential of AI platforms to grow business revenue, the study shows that many businesses face challenges to bring this potential to life.
Lack of specialized skills was the top challenge identified across the region, followed by data foundations lacking sufficient governance processes and a lack of clear evaluation criteria for AI solutions.
Evaluating new tools
These second and third challenges feed into this same idea that knowledge at the leadership level holds great power for a business. Driving appropriate data governance processes and knowing how to evaluate AI’s ability to meet the needs of our business need to be understood at the executive level so we can speak to the value of AI with confidence.
In any business opportunity, the biggest risk can be in not knowing what we don’t know. False confidence and a fear of missing out can be a dangerous cocktail.
We need to build comprehension around the fundamentals of AI in business to build a plan that points our investments toward long-term objectives.
The more knowledge we gain, the better we become at asking questions about the important unknowns and how to require technology partners to give us the insights we need to make informed decisions.
We need to build comprehension around the fundamentals of AI in business to build a plan that points our investments toward long-term objectives.
Seeking an AI strategy
A long-term AI strategy must consider our whole business ecosystem before we even begin integrating AI into it. In the absence of this vision, AI investments can simply add cost but no measurable value, and poor choices in AI tool sets could lead to unintended reliance on specific vendors or new layers of tech debt.
It’s a reality for some organizations today that their early AI efforts are delivering poor results due to limited maintenance capabilities. If models are drifting, they can find themselves giving up on the powerful data-driven insights that well-managed AI has to offer. The moment you lose trust in your AI, it is difficult to recover.
A long-term AI strategy must consider our whole business ecosystem before we ever near integration of AI into our business systems.
Trust is at the heart of successful AI integration. Trustworthy AI requires careful integration with business data, avoids external influences and biases, and is constantly managed through a ModelOps solution to monitor for drift, hallucinations and for ongoing assessment of efficacy and efficiency.
These are managed at the technical level but must be defined by the executive as essentials of maintaining trust and pursuing long-term value.
Our research shows that organizations demonstrating good AI leadership are solving the skill gap differently to those who are following behind. AI followers are more likely to rely on external consultants and suppliers to deliver AI using off-the-shelf models, but then they are also more likely to be doing more code-centered work internally.
Customization for value creation
AI leaders are putting more emphasis on building custom models for their business. They then maximize low and no-code platforms in ModelOps to spread AI capability across the organization.
This reduces the demand on data scientists by making tools more accessible for non-technical teams while creating more opportunities for AI to generate new value in more ways. With carefully designed foundations in place, AI can be unleashed.
When the decision-makers know how to build a robust AI strategy, the technical have the greatest chance possible of succeeding within any business.
I know firsthand that, even as a tech industry executive, some focused training has elevated my understanding around AI dramatically. SAS has also worked with executives on coaching workshops to give them the fundamentals they need to analyze the future of AI in their businesses.
Elevating executive comprehension on AI fundamentals, as well as its risks and opportunities, may be the best skill gap to close. When the decision-makers know how to build a robust AI strategy, the technical have the greatest chance possible of succeeding within any business.